How Textio builds responsible AI
Textio was the first to market purpose-built AI for HR and it’s the reason we are trusted by leading organizations across the world today. AI details, safety, and quality matter to us, because the real-world outcomes matter to us.
Here’s a little more about the ways we ensure safety and intended results with AI.
Building for a specific purpose, and with bias in mind
AI that isn’t built with bias and purpose in mind from the start will produce biased outcomes. This is already happening—broad biases are baked into training data and widely available AI tools because they already exist in the real world.
At Textio, we design purpose-built tools, meaning we create AI for a specific purpose—like feedback—not as a general tool. This focus allows us to carefully select training data and measure AI performance specific to each use case. Unlike general tools, our AI is trained on targeted data, ensuring quality, relevance, and usability.
From the very first development step, we design with bias in mind. Our multi-layered bias mitigation approach includes evaluating datasets and model outputs to ensure
intended results.
Measuring bias in training data
We account for bias in training data in two ways:
Measuring bias in classification
We use classification to determine the context of a phrase and whether it should be “flagged” according to what you’re writing (employee feedback, a performance review, a job post, etc.). For example, is “bubbly” talking about a person or a soda in the sentence “She brings a bubbly presence to work”?
We test bias in our classification models using an F-score. This measures how well the model finds the correct answers and how many correct answers it misses. If we test our model over the same data with different names (“Sally is a bubbly person” and “Bob is a bubbly person”), we should see consistent F-scores. If the F-scores are not consistent, there is bias.
Measuring bias in generative AI
Textio’s generative AI features take input text or answers to prompts and can write, rewrite, or expand the message. One way we measure bias in these features is to vary names in the input text and test if the generated content is different. For example, we’d test “Rewrite: Sally is a bubbly person” and “Rewrite: Bob is a bubbly person” and compare the results.
The challenge is that generative AI models will give different answers to the same text each time you ask. So how do we know that the variation in the output we’re seeing is because of demographic biases (age, race, etc.)?
To determine whether the differences are meaningful across demographic groups, we collect different generative AI outputs for each variation (for example, male vs. female names) at a large scale. We then run a statistical model to compare the distribution of words across each of these groups. If there is a significant difference in the language used in one group over another (defined by the p-value, where p < .05), we can confidently say the output of the generative AI model is biased. If so, we would then:
- Do a qualitative analysis of the bias to identify the themes and characteristics of the differences
- Iterate on the prompt strategy and add hard-coded rules (if necessary) to correct the behaviors of the AI
- Remeasure
Ongoing monitoring and evaluation
We monitor the health of our models and mitigate the potential risk of “drift”—changes in the model’s expected performance—by tracking the appearance and removal rates of “highlights”
(which is how phrases are “flagged” in Textio), as well as the acceptance rates for our suggestions.
If we have a feature with a low removal rate, we label a new dataset and evaluate how well the existing model is performing over this new data (using the F-score statistic). We can improve the model further by collecting new data, labeling, and re-training the model.
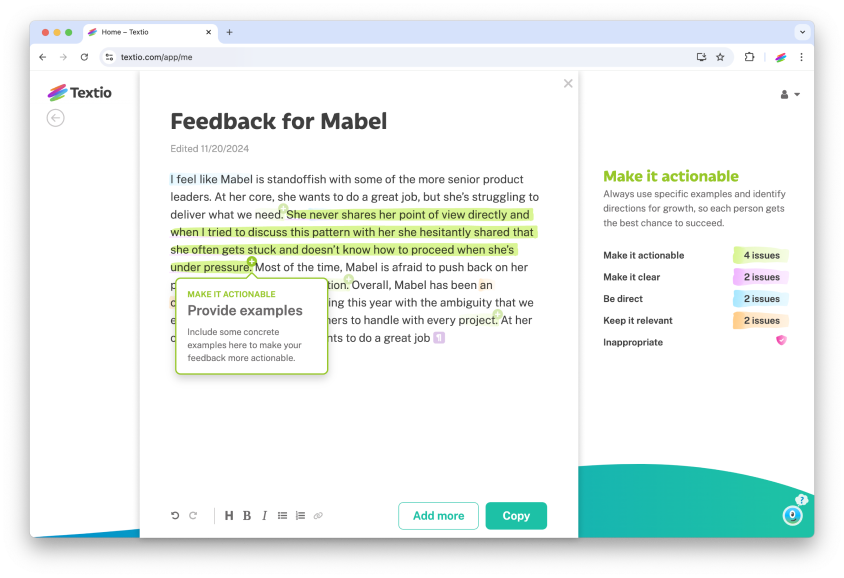
Why Textio is the team to lead responsible AI development
Many people know Textio for our long-time work in bias in language. But even those who know us don’t often realize the unique expertise we have on our team. They also don’t typically see the lengths we go to in terms of representation, inclusion, and belonging—and why DEI on a team creates safer, better products.
Many Textios are experts in their fields. We have PhDs and Masters in areas like Computational Linguistics, Linguistics and Cognitive Science, Speech and Language Processing, and Information and Data Science. We also have language experts in-house who annotate training data.
Additionally, Textio’s Chief Scientist Emeritus and Co-Founder, Kieran Snyder, has a Ph.D. in Linguistics and Cognitive Science and a long career in exploring language, bias, and technology. She is a member of a working group for the National Institute of Standards and Technology (NIST), focused on setting best practices for “developing and deploying safe, secure and trustworthy AI systems,” as ordered by President Biden. She has also advised the Congressional Caucus for AI on national policy.
It’s not easy to develop AI this way. And there are certainly faster ways. But fast and easy are not what powerful tools like today’s AI require. What is required is a deliberate and careful approach. A responsible approach.