AI is transformative.
Let’s be intentional about it.
Beyond the hype is huge potential for AI to make our work and lives better.
When we tune out the marketing noise and focus on purposeful AI initiatives, we stop wasting time and start realizing AI’s promise. Textio is proud to lead responsible AI development through innovation, education, and advocacy.
Pillars of responsible AI
Textio consistently advocates for four key components of responsible AI development in HR.
Responsibility in practice
We take AI seriously. This isn’t new to us, and it’s not a marketing gimmick. We promise and deliver on responsible AI, and this is what it looks like.
How we build our team
Textio has the #1 inclusive recruiting product in the industry. We pair that with a culture of support, belonging, and actionable feedback to build a diverse and empowered AI team.
How we do R&D
We start with “How can we make things better?” not with “How can we add AI?” Our goal is improving work lives, not AI-ifying everything we see.
How we get our data
Your data belongs to you. You’ll never be tricked into training Textio. We source data from case study partners and users.
How we train our models
Textio is known for helping HR teams avoid bias, and that’s for good reason. We have long put in the work to build AI that doesn’t just reduce bias, it also promotes inclusion.
How we guarantee results
Our position is that AI is only useful if it actually makes you faster and better. Textio triple-verifies AI output for accuracy, quality, and safety before you ever even see it.
Understanding what responsible AI looks like
In our Mindful AI video series, uncover biases embedded in generative AI tools with Textio Co-founder and Chief Scientist Emeritus Kieran Snyder. Learn how to spot problematic patterns and what to look for in your search for safe AI for HR.
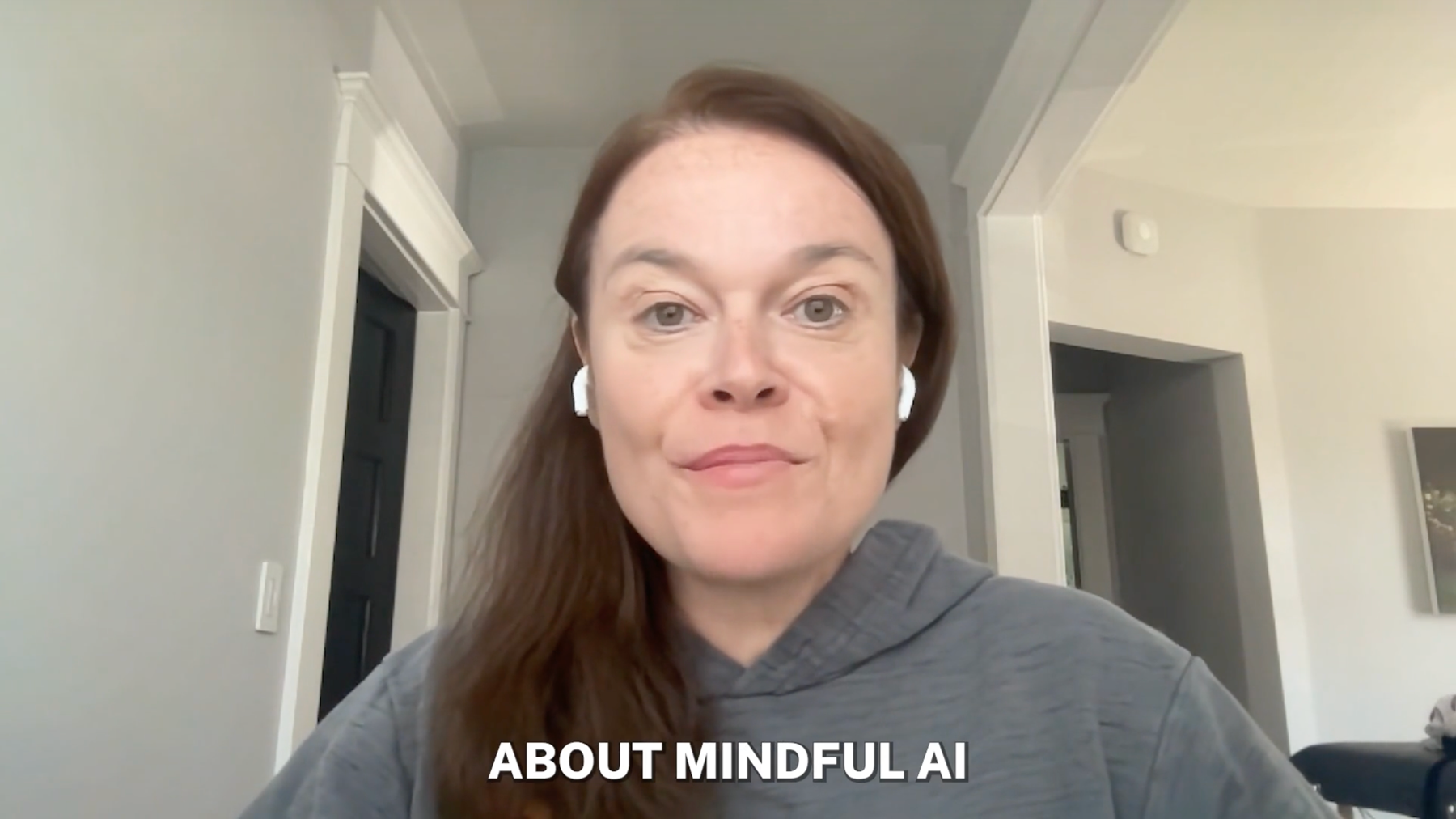
Putting the power of AI to good use
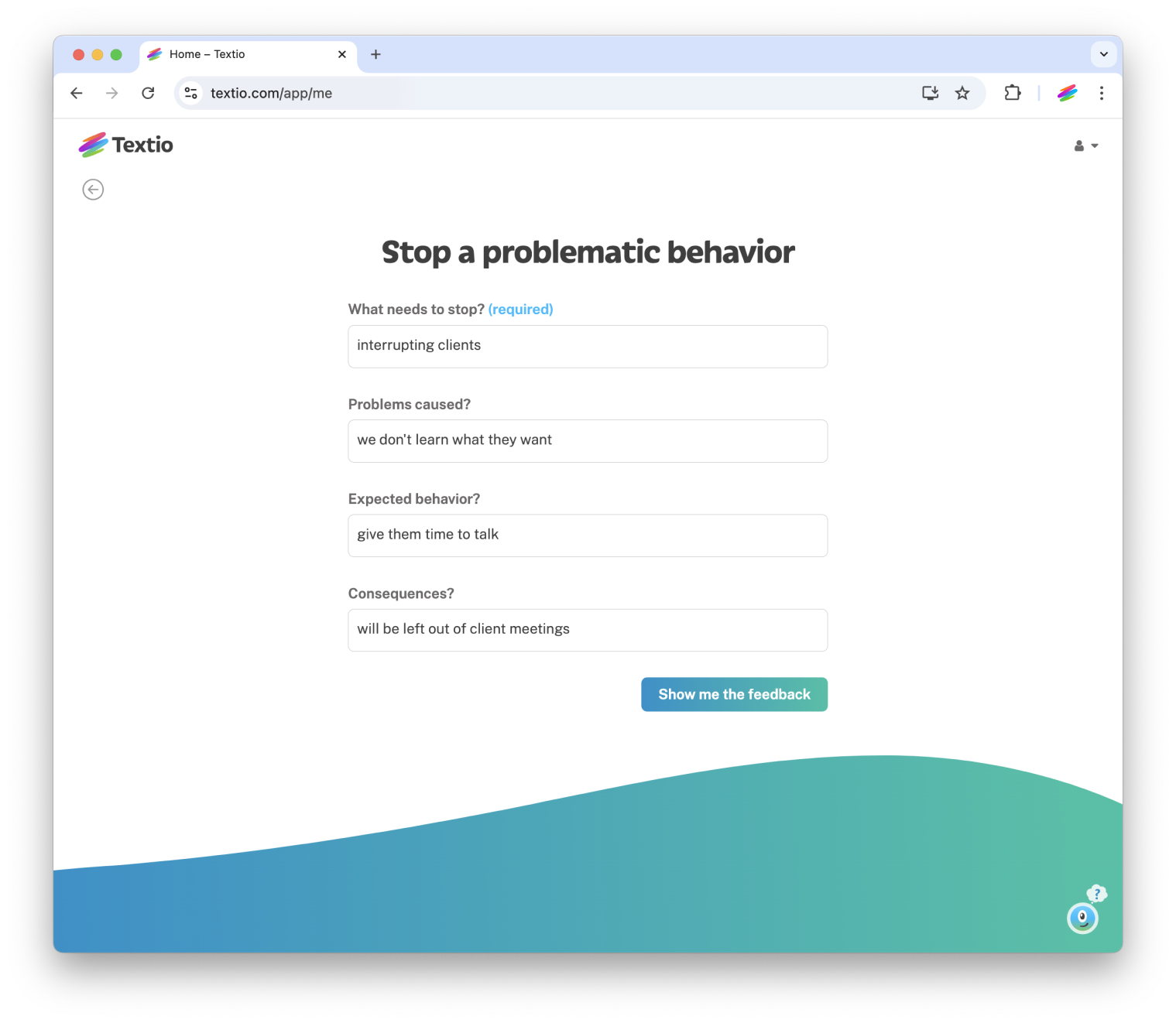
Our vision for the future
- AI is a transformative force for HR, not just speeding up the status quo but actually redefining how HR can support and enable productive teams across the talent lifecycle
- AI development practices are transparent, and HR teams have the tools they need to evaluate AI and make intentional choices when choosing and deploying these tools
- AI is built with bias in mind from the beginning, so it breaks patterns of systemic bias at scale instead of perpetuating them
- AI is secure and respectful of personal and company data
- AI is deployed in a purposeful, responsible way to accelerate specific outcomes, not tacked on as an afterthought
- Textio products and partnerships are making all of these things a reality, one new product at a time
Ongoing research
Textio regularly publishes research on AI and workplace bias to keep our industry’s most important issues top of mind.
Investigating general-use AI
Textio periodically deep-dives into public AI tools to understand the potential for proliferation of bias, harm, and otherwise frustrating AI experiences.
Recent findings:
Building with bias in mind
At Textio, we have several layers of bias mitigation and quality assurance built into our approach from the very first step of development.
Bias creeps into training data two ways: lack of diversity and representation in the training examples; and bias of human labelers.
It’s not unusual for a dataset to be, for example, heavily based on males in their 30s. That’s bias in the representation of the dataset. We mitigate this by balancing our datasets across demographics (gender, race, etc.).
Then there’s the risk of bias in human labelers. Before data can be used for AI training purposes, it must be “labeled” or annotated to show the model how it should be interpreting such data.
The problem is that people labeling data can have their own biases. We assess this by having multiple people annotate the same text and using a diverse group of experts to label our data. We measure how often the annotators agree with each other using Cohen’s Kappa statistic. High agreement (in the 75-80% range) indicates better quality data and assures individual bias is not introduced.
In cases where annotators disagree, we have a separate annotator tie-break before it’s used for our model training. If the agreement is not at least 75%, we retrain the annotators and re-annotate the data.
We use classification—a machine learning method—to determine the context of a phrase and whether it should be “flagged” according to what you’re writing (employee feedback, a performance review, a job post, etc.).
We test bias in our classification models on a common ML metric called an F-score. This is a statistic that measures how well the model finds the correct answers and how many correct answers it misses. If we test our model over the same data with different names (“Sally is a bubbly person” and “Bob is a bubbly person”), we should see consistent F-scores. If the F-scores are not consistent, there is bias.
When we find bias, we identify the source of bias, mediate appropriately, and retest. Some things we consider:
- Is the data balanced? Do we need more representative data?
- Do we need to choose a different model? Or do we need to re-train the model with different parameters?
- Is there bias in the data we use to measure the performance of the model?
- Consider incorporating “in-processing fairness techniques” that influence how the model learns
Textio’s generative AI features take input text or answers to prompts and can write, rewrite, or expand the message. We test to make sure that the output doesn’t contain demographic biases based on gender, race, or age.
One way we measure bias in these features is to vary names in the input text and test if the generated content is different. For example, we’d test “Rewrite: Sally is a bubbly person” and “Rewrite: Bob is a bubbly person” and compare the results.
To determine whether the differences are meaningful across demographic groups, we collect different generative AI outputs for each variation (for example, male vs. female names) at a large scale. We then run a paired t-test to compare the distribution of words across each of these groups. If there is a significant difference in the language used in one group over another (defined by the p-value, where p < .05), we can confidently say the output of the generative AI model is biased. If so, we would then:
- Do a qualitative analysis of the bias to identify the themes and characteristics of the differences
- Iterate on the prompt strategy and add hard-coded rules (if necessary) to correct the behaviors of the AI
- Remeasure