Can the words you use change the people you work with?
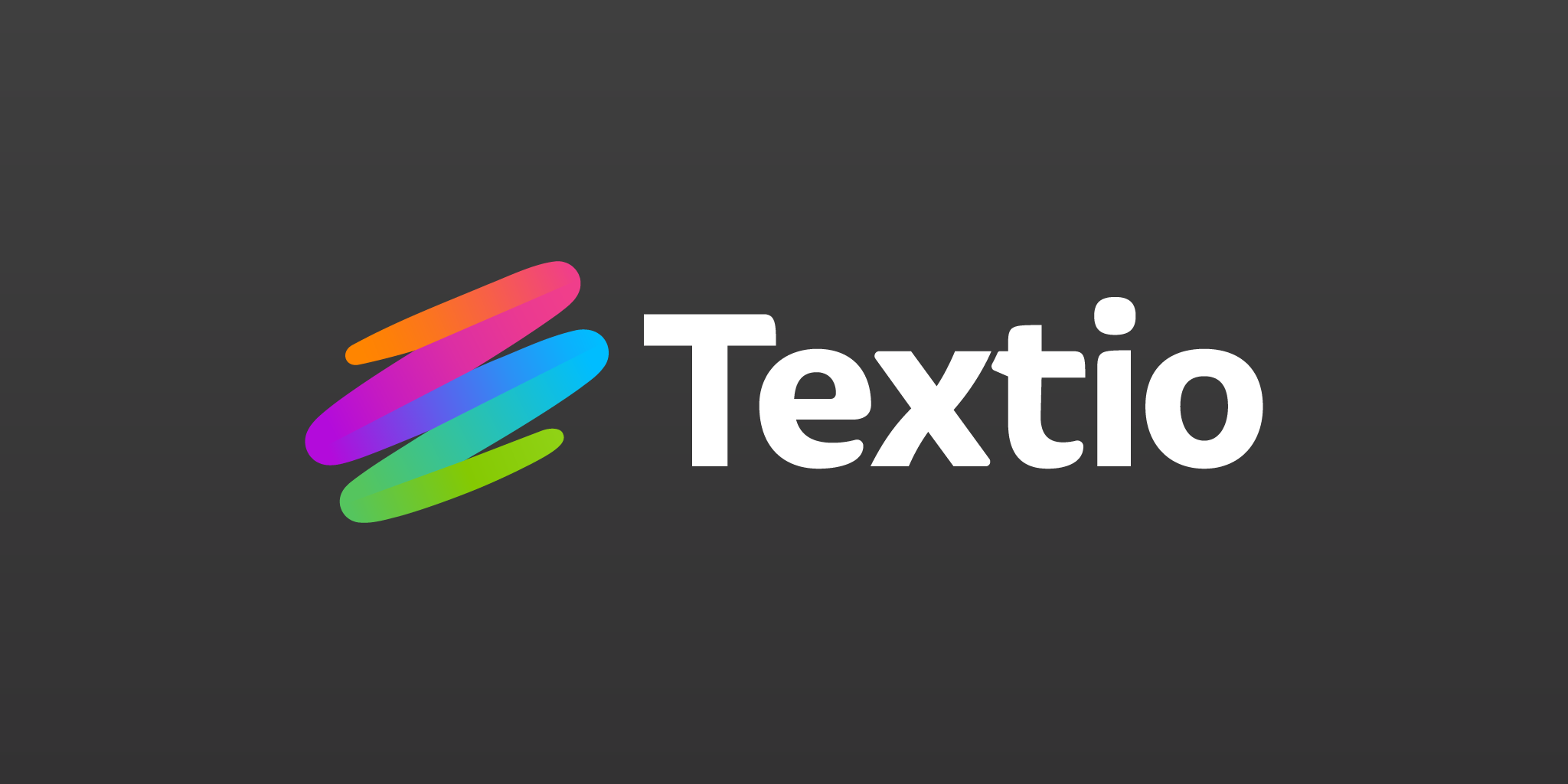
Think about the last time you asked someone to review something important like a resume, housing application, or blog post. Ever practiced a hard conversation in the shower? When we care about how people will respond, we humans have a tendency to finesse every. single. word.
The fact is, every time we iterate on the words we use, we’re just guessing based on our gut or a handful of past experiences. This is an interesting problem to consider for people analytics professionals, or anyone with a large quantity of data at their disposal. For the data rich amongst us, how do we take the guessing out of the words we use?
For the last 1.5 years as a customer success engineer at Textio, I’ve been tasked with measuring how language impacts the way that people respond. Through Data Exchange, Textio receives job text and their hiring outcomes from our customers. In my role I identify key linguistic factors and how they relate to hiring, such as:
As people operations professionals, recruiting and talent teams are incredibly data-savvy. They can spit out their average days to fill, career page visits per month, and applicant stats like *that*. This makes them a rather skeptical and inquisitive bunch, and there’s real pressure on SaaS offerings like Textio to see and prove results.
But while most companies are well-versed in their own people analytics, most have never measured language before. Conveniently, I have a background in linguistics that makes this fun an ideal challenge.
Finding relationships in language
A recent analysis of Expedia’s hiring data helped illuminate what goes on behind the scenes during a linguistic analysis at Textio. The big question at hand: When a customer sees their hiring results change, how do we know it’s because of the language they used?
Job descriptions are one example of a place where we use language at work and track enough relevant data to measure cause and effect. For example, Expedia’s hiring data showed a clear relationship between how biased a job was (masculine or feminine in tone) and how long it took to fill. When the language in their jobs is more inclusive, Expedia finds the right person and fills the role quickly:
To drill into how we know that words impacted the people who applied, let’s look at a case where the relationship isn’t so clear.
According to Rupert Bader, Expedia’s senior director of people analytics, the Expedia team is keen to make their workforce more balanced and better reflect their customers. Women make up around 25% of the engineering workforce at Expedia. As early adopters of Textio, Expedia’s technology team has been very intentional about their gender tone, targeting a neutral-to-feminine tone for the technology jobs they wrote in 2017.
Compare this to Expedia’s other highest-volume job function, Partner Services. A little more than half of all applicants to the Partner Services Group are women. It wasn’t a priority for Expedia to target feminine tones for these jobs (which were already gender-neutral on average, according to Textio’s prediction on how the words would perform). But when you look at the trendline across the data without the historical context for how each group performs, it looks like feminine tones cause fewer women to apply.
This is just one example of how companies constantly evolve their hiring processes in ways that skew their data. We hear lots of nitty gritty questions about this: What if you sponsor a women-in-tech conference while the job is posted, leading to an influx in women applying? What if hiring managers are slammed, and candidates who would normally make it to the screening get passed up? The what-ifs go on and on.
These are very real questions. To ensure Textio’s augmented writing model is accurate, we use statistical tools to isolate the impact of language across all of Textio’s data.
So how do we block out the noise?
Congratulations! You’re about to skip Statistics 101 and jump directly to statistics 102.
Let’s go back to our initial question: When Textio measures your hiring results, how do we isolate the impact of language from all the other factors? We can use statistical tools like multiple regression to control the effects of certain variables and measure the one we want to evaluate. Let’s look at a non-linguistic example first.
Imagine you and your friends live in The City. You all have different salaries and prefer to live in different neighborhoods. You want to predict how much your friends spend on rent based on where they live and how much they make.
It’s easy to imagine how salary independently influences rent. As someone’s salary increases, you’d predict they’re willing to pay more for rent regardless of the neighborhood. Neighborhood choice can also independently influence rent — for example, someone could like living outside of the city where rent is cheaper, regardless of how much they make. Finally, neighborhood and salary can work together. Some of your friends prefer to live in a hip place, but can only afford to live in certain neighborhoods based on how much they make. That’s neighborhood choice “interacting” with salary to determine rent.
Hiring data yields nearly endless potential interacting factors. To isolate just the language, we need to identify where different variables affect the outcome we want to track more than others. This is a good time to introduce the idea of confounders:
Expedia’s Technology group is a great example. Technology jobs are more likely to be feminine because of Expedia’s writing and hiring goals. They’re also historically more likely to attract more men than women. We want to understand the effect of language (in this case, gender tone) on the percent of women who apply regardless of all the other potentially confounding factors.
We can use multiple regression to isolate this relationship. First we assess all the confounding factors. How does job family impact the percentage of women who apply? What about where it was posted, or when it was posted? Once we understand how much variation lies within each of those individual relationships, we can statistically remove the variation and hold them constant.
If that seems abstract, remember that even everyday statistics rely on predictions and hypotheticals. When you buy car insurance, the insurance company doesn’t know whether or not you’ll get into an accident. It calculates all of the potential risks based on your age, gender, location, and more. None of these seem relevant to driving a car, but they’re potential confounders which allow the insurance company to set your premium by better predicting the risk that they’ll need to spend money on you.
Once we’ve held the effects of external variables constant, we can examine the partial effect that one particular variable like gender tone has on a hiring outcome. Expedia sees 8% more women apply when jobs are feminine vs. masculine in tone when confounders such as job type were held constant.
So to summarize, here are the steps we’ve covered so far:
- Identify the important factors affecting the data
- Measure how each factor affects our outcome, and how much
- Statistically remove this variation to focus on just the thing we want to measure (e.g. language)
So how does Textio work these principles back into its massive dataset and the guidance you see? We can’t deal in only hypotheticals, or Textio (and statistical analysis in general) would be useless. Knowing how external factors impact customer results, we can measure how words work across all of the data. For instance, job type has a very real impact on which words work well for your intended audience.
Take another Expedia example: How does language impact time to fill? Across Expedia’s data, Textio measured certain words that caused jobs to fill more quickly or more slowly regardless of job type. The platform couldn’t do that without knowing for certain all of the variation across confounding variables is considered.
When they write in Textio, Expedia users get to see the company-specific words that impact their hiring results in addition to the usual orange and green phrases you see. No one else sees them, because Textio has discovered that they are uniquely impactful at Expedia. When a pattern shows up in enough companies’ data sets, it gets added to Textio’s broader writing guidance.
Want to know what your unique language patterns are doing to your hiring results? As a customer success engineer, there’s one major takeaway I’ll leave you with when it comes to measuring language: The more visibility and data you can provide into all of your potential confounding factors, the better. If you have a background in experimental design, and want to try this linguistic analysis yourself? Come work for us! We’re hiring.