Language in your job post predicts the gender of your hire
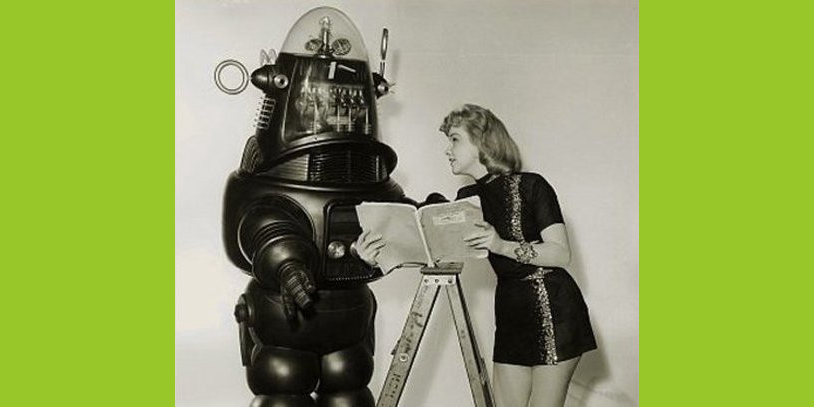
Shortly after founding Textio, I went on a mission to meet other women founding learning loop companies. I found a few on Twitter, but mostly I came up short. Among founders, women are vastly outnumbered to begin with, but this felt like something more.
As Textio developed, I heard similar anecdotal observations elsewhere. The women I met in the field didn’t know many others. The investors I knew were funding machine intelligence companies left and right, but even the firms with several women in their portfolios said they just weren’t getting pitched by women in this space.
Last December, I spoke at a machine intelligence conference. Check out the speaker list, which includes just three women out of 39 speakers: one reporter, one investor, and me. One of the most prominent machine intelligence events in the industry came up with exactly one female founder to include.
This got me thinking: Is this just business as usual in technology, or does machine intelligence have an even more pronounced gender gap? And what would Textio say about it? Do machine intelligence jobs show greater bias than others in the industry?
To understand the results, let’s take a quick detour to talk about how Textio calculates bias.
A language pattern is considered gendered if it statistically changes the proportion of men and women who respond to a job post containing it.
To figure out which language is gendered, Textio uses outcomes within our customers’ hiring data. If a particular phrase predicts a high proportion of women applying, the phrase is considered feminine-tone. If it predicts a high proportion of men applying, the phrase is considered masculine-tone.
For every job post that Textio reads, the job is assigned a bias score according to the presence or absence of gendered language patterns in the post. Because the patterns are statistical and quantitative, they predict the gender of the people who are likely to apply (which, of course, has a big impact on who you hire).
Check out these findings from our enterprise clients: In jobs where a man is hired, the original job post averages almost twice as many masculine-tone phrases as feminine. In jobs where a woman is hired, Textio finds the exact opposite: twice as many feminine-tone phrases as masculine in the job post.
In other words, the bias in your original job post impacts who you’re going to hire. This makes sense; the language you use changes who applies to your job, and you’re much more likely to hire a woman into a tech role if your pipeline has several women to consider.
Textio recognizes tens of thousands of permutations of gendered language, and the patterns change all the time based on what’s happening in the market. Bias trainings and phrase checklists don’t make much real difference to who you hire, but data always wins.
For instance, common phrases that exert a bias effect that don’t show up on any qualitative checklists include exhaustive, enforcement, and fearless (all masculine-tone) and transparent, catalyst, and in touch with (all feminine-tone). You can only find these patterns by measuring over time with an enormous data set of real hiring outcomes — exactly what Textio’s engine is designed to do.
Textio compares the language in each new job to the language of other similar jobs where applicant demographics are known. Based on similarities and differences, Textio then assigns each job a bias score between 1 and -1, depending on the overall tone of the listing. Bias scores that are between 0 and 1 are considered feminine, and scores between 0 and -1 are considered masculine.
With that in mind, let’s return to the machine intelligence question. What does Textio say about bias in machine intelligence jobs compared to others in tech?
For this analysis, we took over 78,000 engineering jobs that Textio’s predictive engine has closely classified over the last couple of months. They fall into seven distinct categories:
- Machine intelligence
- Data science
- Front-end
- Back-end
- Full-stack
- General software engineer
- Research engineer/scientist
Here’s how the average bias scores break down across the 78,768 listings included in the analysis:
In other words, machine intelligence jobs aren’t just the most masculine in tone. They’re the most masculine in tone by a really wide margin.
Textio’s predictive engine shows that the use of gendered language in your job post impacts who is going to apply. In light of the bias distributions above, the apparent scarcity of women in machine intelligence jobs is probably more than anecdotal.
This raises so many more questions, like where are all the women I knew in grad school, and why aren’t they working in the industry? But in the meantime I’ve concluded that it’s not just me: machine intelligence has a bias problem. And given the enormous financial opportunity that learning loop companies are sitting on, it’s a bias problem with vast cultural ramifications.
If you’re wondering how your own machine intelligence jobs stack up, find out what Textio has to say. And if you’re a woman who’s founded a learning loop company, I’d love to meet you.