Mindful AI: Understanding training data and bias
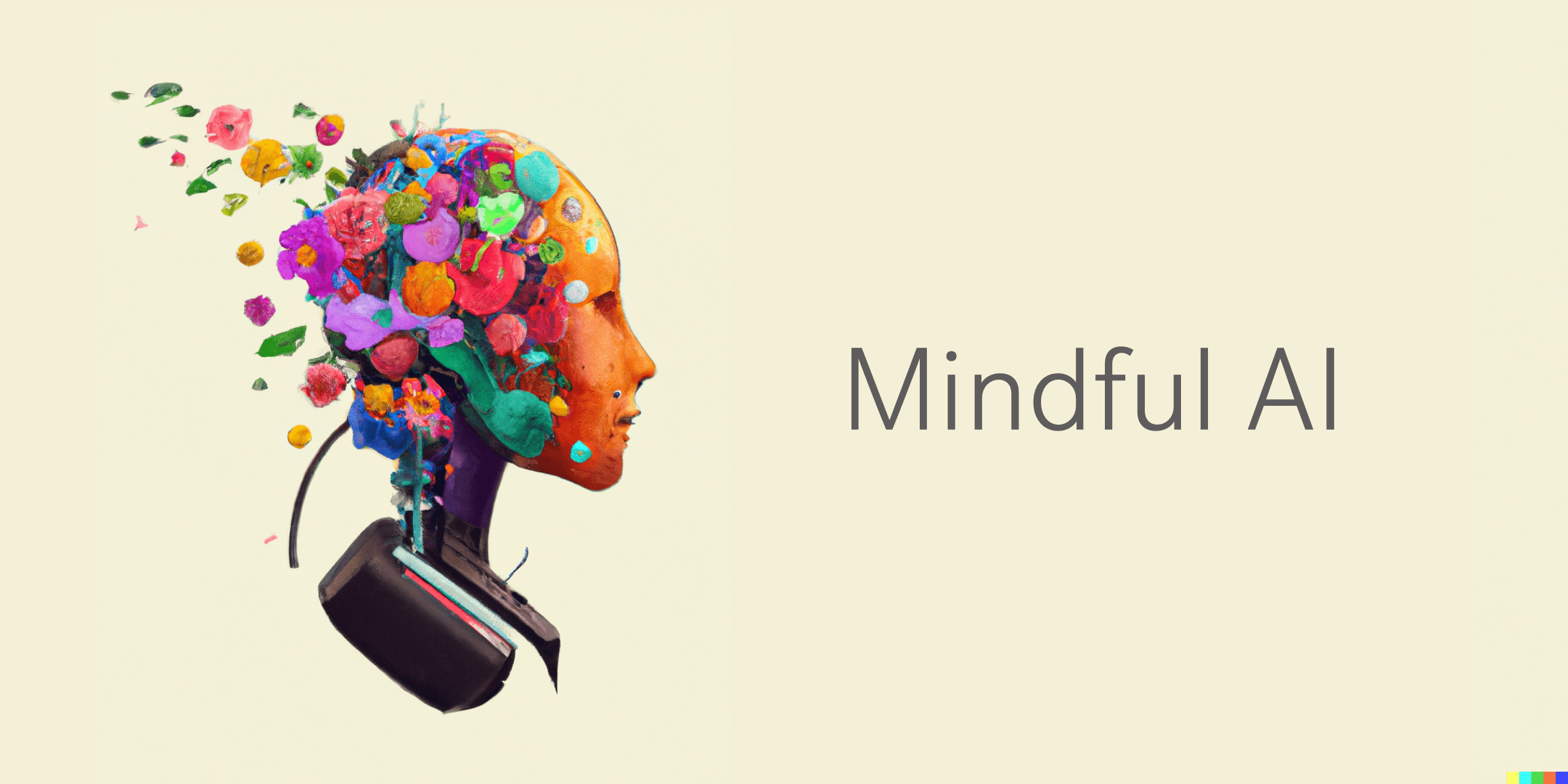
Generative AI has the power to transform the modern workplace in countless ways. It also carries significant risks. One of the main concerns with generative AI is its potential to reinforce existing patterns and biases. And when it comes to the modern workplace, we are biased by default.
Not so sure? The numbers speak for themselves. While white men make up a third of entry-level jobs, they hold over 60% of C-suite positions. Meanwhile, women of color account for just 19% of entry-level jobs, and a mere 5% of C-suite positions.
At its core, generative AI is essentially a technologically advanced mirror. What goes into it is what comes out, and if the inputs are biased, the outputs will be too.
But what if we could use generative AI in a way that didn’t propagate this kind of harm? Even further, what if we could use generative AI to actively combat bias rather than reinforce it? In this series, we’re going to talk about how even non-experts can use AI to create positive rather than negative outcomes.
When it comes to using generative AI, our minimum bar is that we shouldn’t be perpetuating harm. But as we’ll discuss later in this series, we can also go further than that: We can make generative AI an active ally in the DEI revolution.
This week, we’re going to start with the table stakes: not perpetuating bias. So let’s talk about training data.
The meal is only as good as the ingredients
Any good cook will tell you that it’s easier to cook something good with farm-fresh ingredients than with stuff out of a can. Of course, good ingredients alone are not enough; it’s still possible to cook them wrong. But when the ingredients are high-quality, it’s much easier even for a novice cook to make a great dish.
Training data, or the extremely large data sets that are used to teach an AI model how to make predictions, is like the ingredients for your generative AI meal. The final dish depends a lot on the ingredients you‘re able to include. When you have good ingredients, you can make a pretty good meal. With so-so ingredients, it’s much harder.
Unfortunately, most off-the-shelf generative AI tools (including ChatGPT) are built with a mix of good and bad ingredients, so the output can be problematic. For instance, when ChatGPT is asked to write sample performance reviews for a range of occupations, the output shows significant gender bias.
Gender stereotypes that appear in performance reviews written by ChatGPT
In this case, ChatGPT perpetuates the biases that are in its underlying training data. When most or all of the training text about kindergarten teachers and receptionists is written with she/her pronouns, ChatGPT’s output will default to using these pronouns as well.
By default, generative AI learns the biases of its training data. To change the final dish, we’d have to change the ingredients; in this case, we’d need to intentionally include more training text showing different gender patterns. This is not easy to do in a scaleable way. After all, the training data shows bias because people show bias too. For the teams making the core generative algorithms that everyone else is using, this is a critical priority.
What if you can’t choose the ingredients yourself?
Most likely, you aren’t building your own core generative algorithms or training data sets. If you’re like most people, you’re not developing these products yourself; you’re just trying to use them in the course of your normal work. In other words, when you prep your meal—like when you ask ChatGPT to write your job description, draft your tweets, or write an email on your behalf—you’re at the mercy of whoever stocked the kitchen.
This makes it extra important that you choose your task wisely. Assuming you care about the quality of your output, generative AI training data is simply better suited to some kinds of tasks than others. Asking ChatGPT to write a totally original document? Prepare for the worst: gender bias, racial bias, age bias, and more. (And whatever you do, don’t ask ChatGPT to be funny.)
But that doesn’t mean generative AI can’t provide enormous value in net positive ways. The key is to pick tasks (and AI products that are specifically designed to help with these tasks) thoughtfully, so the training data is designed for the task at hand.
For instance, we build Textio Lift to help people write performance reviews and other feedback at work. One of our most important generative AI features is very straightforward: Textio Lift detects when you’ve written sentences that are too complicated or convoluted, and it simplifies them for you with one click.
This sentence is too complicated
This rewrite is a lot easier to understand!
In this case, Textio starts with your own content and rewrites with a single click so that it is easier for the reader to understand. The underlying training data is perfectly designed to be great for this task. A large corpus of well-constructed sentences is a great training set for turning complex writing into something simpler and clearer. In this case, no novel content is getting generated, so there’s limited opportunity to introduce new false harmful content (sometimes called hallucinations). Like any good editor, this feature helps you say what you meant to say all along—just much more clearly.
Large Language Models (LLMs) are great for simplifying and summarizing
Mainstream public conversation about generative AI has largely focused on writing new documents. Millions of people have tried ChatGPT for this purpose. Citing plagiarism concerns, numerous school systems have forbidden student use of ChatGPT. Several software vendors have embraced this use case, focusing on spitting out plausible-sounding (but not necessarily high-performing) content quickly.
This kind of write-from-scratch scenario is also the riskiest when it comes to hallucinations—generating content that sounds plausible, but is actually false or otherwise harmful. These risks can be managed, and we’ll talk more about how in an upcoming article. But without taking intentional steps to mitigate the risk, the odds of ChatGPT and other generative AI tools writing problematic content are quite high.
However, there’s a large set of scenarios for generative AI that are more like the Textio Lift feature described above. In these scenarios, generative AI is used to simplify, summarize, or restate text so that it’s easier to read or otherwise work with. These are lower-risk use cases because LLM training data is uniquely well-suited to enabling them. The task at hand is not to write something brand-new, but to state something that’s already been said more clearly.
For instance, one of the most common use cases is in meeting summarization. Otter.ai aims to provide meeting summaries so that you can know what was said during a meeting without having to listen to the recording or read an entire transcript; it also pulls out key follow-up items from the discussion. Gong provides after-the-fact call summaries that rely on generative AI behind the scenes, and Zoom IQ automatically sums up the contents of a meeting in real time so you can catch up quickly if you join late.
Similarly, a number of information management products are investing in smart summarization. These go way beyond word clouds and sentiment tools. For instance, both Atlassian and Microsoft recently announced tools that offer customers specific insights about how teams are working together. Without being in every conversation yourself, you can see and understand how well the social graph in your organization is working.
Underneath the various use cases, these products and features are all based on the same premise: Generative AI is excellent at distilling a large amount of text down to its essence. And when you ask generative AI like ChatGPT to summarize rather than write from scratch, you’re much less likely to introduce new vectors of harm.
What talent leaders need to know
For any AI tool you’re considering using, ChatGPT or otherwise, you need to ask yourself and your vendors: What’s in the training data behind this product, and what is it designed to be great at? If you’re writing job posts, emails, or other work documents with these tools, your output will propagate all the systemic biases in gender, race, age, and more that show up in the training data. You’re going to need good tools and processes to catch these issues before you publish your document.
Conversely, several of the use cases for generative AI we’ve been discussing here let us work better and faster. They are simple and significant wins for productivity. They don’t create new harmful or biased output, which is a good start.
But we can go further than that: We can use generative AI to subvert the status quo and create real advances in DEI. Next week, we will look at how. We’re going to turn from training data to prompt generation—going beyond ingredients and looking at the skill of the chef.