Three questions you should ask before investing in “AI” for HR
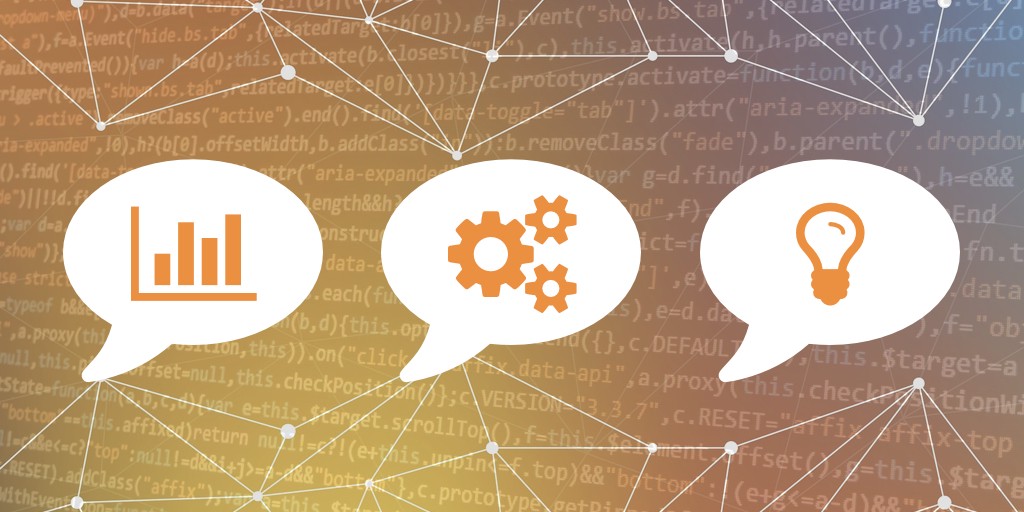
Go to the demo floor of any major HR tech conference these days, and you’re likely to encounter a similar scene: row after row of vendors talking about AI and machine learning and how their version of the technology will help you hire better.
Some of these are brand-new products. Chatbots that interact with your job applicants and employee engagement survey tools based on proprietary research frameworks are popping up everywhere. Many old products are trying to embrace the new tech wave too. It’s hard to find an ATS or a sourcing product that isn’t talking up its AI capabilities.
The landscape is dense enough with AI tag lines that even where the technology delivers results, it can be hard to separate the products that work from the empty promises. Even if you’re an experienced talent leader, it’s tough to know where to begin.
Unfortunately, ignoring the trend isn’t really an option for seasoned talent leaders. Because frankly, even with all the technological and system advances of the last 20 years, hiring is still hard — and if you want to keep growing your business, hiring is still the most important thing your company does. For every person you’re eager to hire, ten other companies are chasing them too. If just one of those companies makes the right tech investment that gives them even a small edge in the battle for talent, you’re at a real disadvantage.
So if ignoring the crowded landscape isn’t an option, how do you make sense of it all? There are three key questions that can help you separate the winner from the wanna-be. Before you make any purchase, make sure the answers to these questions are rock solid.
1. Does the solution promise you a specific, measurable result?
The opportunity offered by learning loops is enormous. With the right data set, machine learning technologies can discover patterns of real predictive significance: which candidate profiles are most likely to work out in your organization, which language is going to attract them, which people are likely to churn out, and much more.
Properly applied, ML-based technology can outperform your personal intuitions and biases. And indeed, every company that promotes its machine learning capability promises better results than you’re getting today.
But how specific are those promises, and do they have customers with clear results? If a product offers you faster hiring, how much faster? If it guarantees a higher response rate from sourced candidates, how much higher? If it promises a more diverse candidate pool, how many new candidates will you see from underrepresented backgrounds, concretely?
When AI or machine learning is the foundation behind a product, the promises that the product makes should be specific and measurable. You’re allowed to — and should — ask for a number you can measure and case studies that illustrate the results. The whole point of these technologies is that they can be specific and predictive in a way that human intuition on its own cannot be.
If someone who talks up their AI tech won’t promise you specific numeric results, they may have a useful product in other ways, but you’d best proceed cautiously when it comes to their AI implementation. The power of these technologies is in the numbers.
2. Was the solution designed around AI from the beginning?
For decades, science fiction writers and Back to the Future fans alike have dreamed of flying cars. A couple of people have even succeeded in building them. But no matter how clever the design, flying cars can’t fly as fast or energy-efficiently as an airplane. Cars don’t make good planes because they were never designed to fly in the first place.
This is exactly what happens when old solutions bolt on AI features to try to stay competitive. Just as you can’t simply take a car and bolt on some jet engines and expect a plane, you can’t take an aging piece of software and bolt on some machine learning code and expect something modern. It was designed for the way you worked ten years ago. It wasn’t designed to be modern.
Only HR tech solutions that are based on machine learning from the beginning can make you a concrete, measurable promise about the improvements you’ll see in your hiring process when you use them. Solutions that weren’t designed with the right data loops and learning technology from the start don’t make measurable promises. They just weren’t designed to.
3. How is the solution different from everything else you’re seeing?
Let’s say you’ve spotted a product that makes a strong measurable promise, and it’s been built with AI and learning loops in mind from the start. There’s one more thing to consider: Does the product solve your problem better than the alternatives? For any solution you’re considering buying, the vendor should be able to tell you what makes them different from the competition.
You have a limited budget, and for every solution you invest in, you want to be confident that the investment gives you a concrete advantage in the talent market. If you see 20 products that all offer the exact same thing, then find the 21st — the one that solves the problem in a new way that can give you a talent advantage.
Hundreds of solutions, but only three questions
The next time you’re at a talent or HR tech conference and you find yourself walking around the demo floor, take some time to consider the solutions you’re seeing with these three questions in mind:
- What specific, measurable promise is this solution making me?
- Was this solution designed with AI from the beginning?
- How is this solution different from everything else here?
If you don’t get good answers, keep walking. If you get great answers, you might just be on to your next strategic talent advantage.